技術:AIが「グランツーリスモ」のチャンピオンドライバーを追い越した
Nature
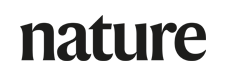
ライバル車との接戦を繰り広げる自動車レースゲーム「グランツーリスモ」で、世界チャンピオンレベルのプレイヤー相手に勝利した人工知能(AI)エージェントについて報告する論文が、Nature に掲載される。このエージェントは、レースのエチケットを守りながら、卓越した走行速度、車両制御、戦術を披露した。今回の知見は、自律航法やAIの基礎研究に役立つかもしれない。
AIの適用可能例の多くでは、人間と関わり合いながら、物理系においてリアルタイムで意思決定を行うことが求められる。自動車レースは、そうした状況の一例だ。ドライバーは、静止摩擦限界で車両を操作しつつ、複雑な戦術的操作を実行して、ライバル車を追い越したりブロックしたりしなければならない。プレイステーション用ゲームの1つである「グランツーリスモ」のようなレースシミュレーションは、実際のレースカーの制御の課題を再現しており、機械学習にとって高難度の適用例になっている。
今回、Peter Wurmanたちは、深層強化学習を使って、Gran Turismo Sophy(GT Sophy)と名付けたAIエージェントに、「グランツーリスモ」をプレイする方法を教えた。GT Sophyは、レースコースで用いる効率的な加速と制動の技術を習得するよう訓練され、さまざまな状況下、または相手にブロックされたときに別の経路を見つける方法を学習した。高性能のAIの訓練で最も困難な側面の1つは、レースのエチケット(外部の人間の審判が決めた一連の緩やかな規則)に違反することに伴う罰則を確実に回避することだ。GT Sophyは、3通りの車両(時速300キロメートルを超える車両を含む)と自動車レース場の組み合わせによって異なるレース課題が提示された状況下で、世界最高のeスポーツドライバー4人と大接戦を演じ、勝利を収めた。
競争的課題(チェス、ポーカーなど)でコンピューターが最強の人間を打ち負かすという流れが続いているが、今回の結果は、この流れを引き継ぐ新たな一歩だ。Wurmanたちは、今回の知見は、自動車レースゲームの楽しさを向上させるだけでなく、プロのドライバーの訓練や新しいレース技術の発見のための現実的でハイレベルな競争状況を作り出すかもしれないと示唆している。また、この手法は、現実世界のシステム(ロボット、空中ドローン、自動運転車など)にも適用できる可能性がある。
An artificial intelligence (AI) agent that can outcompete world champion-level human players in the head-to-head car racing game Gran Turismo is reported in a Nature paper. The agent demonstrated exceptional driving speeds, car control and tactics whilst maintaining racing etiquette. The findings could have useful applications for autonomous navigation and fundamental AI research.
Many potential applications of AI require making real-time decisions in physical systems whilst interacting with humans. Car racing represents an example of these conditions; drivers must execute complex tactical manoeuvres to pass or block opponents whilst operating their vehicles at their traction limits. Racing simulations, such as the PlayStation game Gran Turismo, reproduce the control challenges of real race cars and provide a challenging application for machine learning.
Peter Wurman and colleagues taught an AI agent, named GT Sophy, to play Gran Turismo using deep reinforcement learning. The agent was trained to master the art of accelerating and braking efficiently over race courses, and learned how to find alternative paths in different conditions or when blocked by opponents. One of the most challenging aspects for the training of a successful AI is to ensure it avoids incurring penalties associated with breaching race etiquette, a set of loose rules adjudicated by external human judges. GT Sophy was able to win head-to-head competitions against four of the world’s best e-sport drivers using three car and track combinations, each posing different racing challenges, including vehicles that can reach speeds in excess of 300 km per hour.
These results are another step in the ongoing progression of competitive tasks that computers can beat the very best humans at, such as chess and poker. The findings could make racing games more enjoyable and provide realistic high-level competition for training professional drivers and discovering new racing techniques, the authors suggest. The approach may also find applications in real-world systems, such as robotics, aerial drones and self-driving vehicles.
doi: 10.1038/s41586-021-04357-7
「Nature 関連誌注目のハイライト」は、ネイチャー広報部門が報道関係者向けに作成したリリースを翻訳したものです。より正確かつ詳細な情報が必要な場合には、必ず原著論文をご覧ください。