【人工知能】全くのゼロから独学で囲碁を習得する「アルファ碁ゼロ」
Nature
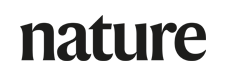
コンピュータープログラム「アルファ碁」の新バージョンである「アルファ碁ゼロ」は、人間による入力をせず、全くの独学で、古典的な戦略ゲームである囲碁を白紙の状態から急速に習得できることが分かった。その上アルファ碁ゼロは、旧バージョンのアルファ碁(2016年3月に囲碁チャンピオンのイ・セドルを破った)との対局で100戦100勝の成績を挙げた。この新しいコンピュータープログラムについて報告する論文が、今週掲載される。
人工知能の大きな課題の1つは、白紙の状態から難度の高い概念を学習し、超人的な習熟を達成するアルゴリズムを開発することだ。旧バージョンのアルファ碁は、人間の囲碁チャンピオンを負かすため、囲碁の専門家による数百万の指し手を用いた教師付き学習と自己対局(この場合はアルファ碁同士の対局)による強化学習を併用して「訓練」された。アルファ碁の「訓練」は数か月間行われ、複数のコンピューターとニューラルネットワークの「訓練」に特化したチップであるテンソル処理ユニット(TPU)を48個必要とした。
このDavid Silver、Julian Schrittwieser、Karen Simonyan、Demis Hassabisたちの研究グループの論文で紹介されているアルファ碁ゼロは、自己対局のみによって学習するため、最初はランダムな指し手で試合を進め、盤面上の位置と囲碁の石だけを入力データとし、人間によるデータ入力は一切なかった。アルファ碁ゼロは、単一のニューラルネットワークを用いているが、このネットワークは、このプログラム自体が選ぶ指し手と対局の勝者を予測するように「訓練」され、自己対局が繰り返されるたびに性能が向上した。アルファ碁ゼロは、1台のコンピューターと4個のTPUを使用している。
「訓練」が数日間続けられ、約500万回の自己対局をへた時、アルファ碁は、棋士を凌駕し、全ての旧バージョンのアルファ碁との対局に勝利した。そして、「訓練」を受けたアルファ碁ゼロは、人間が数千年をかけて概念化した囲碁の原理のいくつかを独自に発見し、歴史の長い囲碁に新たな知見をもたらす新たな戦略を策定するようになった。
A new version of the AlphaGo computer program is able to teach itself to rapidly master the classic strategy game Go, starting from a blank slate and without human input, reports a paper published in Nature this week. The new program, called AlphaGo Zero, defeated its predecessor (which defeated Go champion Lee Sedol in a tournament in March 2016) by 100 games to 0.
A grand challenge for artificial intelligence is to develop an algorithm that learns challenging concepts from a blank slate and with superhuman proficiency. To beat world-champion human players at Go, a previous version of AlphaGo was trained through a combination of supervised learning based on millions of human expert moves and reinforcement learning from self-play. That version of AlphaGo was trained over several months and required multiple machines and 48 TPUs (specialized chips for neural network training).
Here, David Silver, Julian Schrittwieser, Karen Simonyan, Demis Hassabis and colleagues introduce AlphaGo Zero, which learns solely from the games that it plays against itself, starting from random moves, with only the board and pieces as inputs and without human data. AlphaGo Zero uses a single neural network, which is trained to predict the program’s own move selection and the winner of its games, improving with each iteration of self-play. The new program uses a single machine and 4 TPUs.
After a few days of training - including almost 5 million games of self-play - AlphaGo Zero could outperform humans and defeat all previous versions of AlphaGo. As the program trained, it independently discovered some of the same game principles that took humans thousands of years to conceptualize and also developed novel strategies that provide new insights into this ancient game.
doi: 10.1038/nature24270
「Nature 関連誌注目のハイライト」は、ネイチャー広報部門が報道関係者向けに作成したリリースを翻訳したものです。より正確かつ詳細な情報が必要な場合には、必ず原著論文をご覧ください。