Research Abstract
動的ネットワークマーカーによる大きなノイズ下における臨界遷移の早期予兆信号の検出
Identifying early-warning signals of critical transitions with strong noise by dynamical network markers
2015年12月5日 Scientific Reports 5 : 17501 doi: 10.1038/srep17501
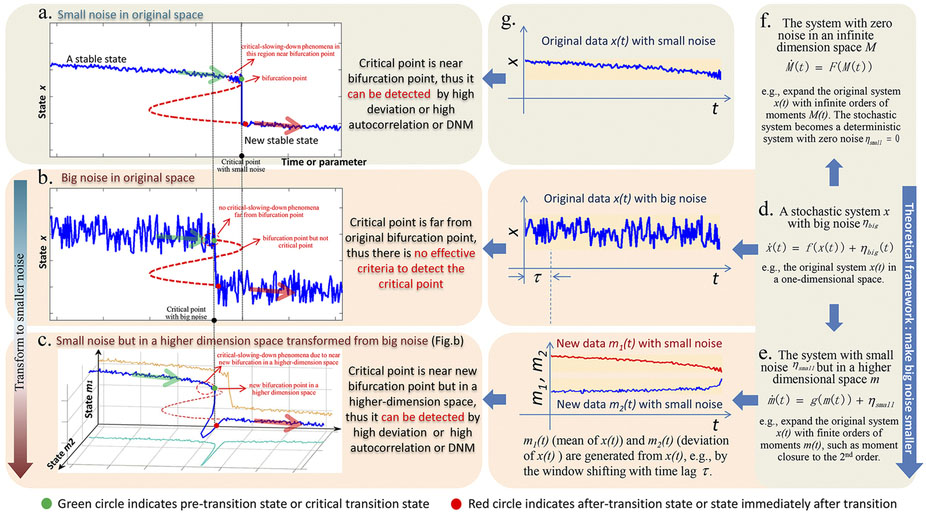
一般に複雑系で臨界遷移の早期予兆信号を検出することは難しい。特にターゲットとなるシステムが大きなノイズにさらされている際は、観察データの強い変動のために従来手法だと失敗してしまう。本研究において、我々はノイズが有意に小さくない時に臨界遷移が古典的な状態遷移でなく確率分布遷移であることを示す。この仮定は現実の系でも広く見られるものである。我々はこのような遷移の前の予兆信号を検出するために、モデルフリーの計算手法を提示している。鍵となる考えは「大きなノイズを小さくしろ」という戦略であり分布埋め込みスキームによる。これは、データを大きなノイズのある観測された状態変数から小さなノイズの分布変数に変換するもので、有意に減少する変動により古典的な判断基準を有効なものにしている。特に、システムを状態ダイナミクス(動力学、動態)から確率分布ダイナミクスに変えるモーメント展開によって観察データの次元を増加させ、より高い次元空間だがはるかにノイズの少ない新しいデータを取り出している。また、我々は変換したより高次元のデータを用いて差し迫った臨界遷移を知らせる動的ネットワークマーカー(DNM)にもとづいた評価基準を開発した。さらに、本手法の有効性を、生物系、生態系および金融システムに関して実証的に示した。
Rui Liu, Pei Chen, Kazuyuki Aihara & Luonan Chen
Corresponding Authors
Identifying early-warning signals of a critical transition for a complex system is difficult, especially when the target system is constantly perturbed by big noise, which makes the traditional methods fail due to the strong fluctuations of the observed data. In this work, we show that the critical transition is not traditional state-transition but probability distribution-transition when the noise is not sufficiently small, which, however, is a ubiquitous case in real systems. We present a model-free computational method to detect the warning signals before such transitions. The key idea behind is a strategy: “making big noise smaller” by a distribution-embedding scheme, which transforms the data from the observed state-variables with big noise to their distribution-variables with small noise, and thus makes the traditional criteria effective because of the significantly reduced fluctuations. Specifically, increasing the dimension of the observed data by moment expansion that changes the system from state-dynamics to probability distribution-dynamics, we derive new data in a higher-dimensional space but with much smaller noise. Then, we develop a criterion based on the dynamical network marker (DNM) to signal the impending critical transition using the transformed higher-dimensional data. We also demonstrate the effectiveness of our method in biological, ecological and financial systems.