Research Abstract
全脳的な内在性回路間の結合パターンから作業記憶の成績上限を予測する
Predicting learning plateau of working memory from whole-brain intrinsic network connectivity patterns
2015年1月5日 Scientific Reports 5 : 7622 doi: 10.1038/srep07622
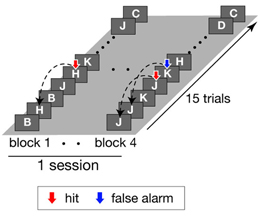
認知機能に関する個人の学習成績は、認知課題の実行中に対応して活動を増す「課題関連」脳領域内の機能的結合に関係する。いっぽう、すべての脳領域は、他の領域や脳全域的な回路網とも結合しているので、学習は、異なる機能を担う回路網間の結合の調節と見ることもできる。そこで今回、学習成績は、課題関連領域と関連の弱い領域とを含めた内在性回路網間の機能的結合によって決まると仮定した。被験者は、まず安静時での機能的MRIを計測し、別の日に作業記憶の短時間(80-90分)の訓練を受けた。その上で全脳的な内在性回路網間の機能的結合パターンを計算し、疎な線形回帰モデルによって個人のパターンから成績の上限を予測できるか否か検討した。その結果、モデルから高精度な予測が可能だった(R2 = 0.73, p = 0.003)。左の前頭-頭頂回路を含む課題関連領域内で正の相関をもつ結合は、予測の約半分(48%)に寄与した。また、上の仮説と符合するように、課題関連領域と弱関連領域との結合も、ほぼ同等な寄与(44%)を示した。今回の知見から、学習成績は、課題関連領域内および課題関連領域-弱関連領域間の、両方のシステムレベルの相互作用で決まる可能性が示唆される。
Masahiro Yamashita, Mitsuo Kawato & Hiroshi Imamizu
Corresponding Author(s)
Individual learning performance of cognitive function is related to functional connections within ‘task-activated’ regions where activities increase during the corresponding cognitive tasks. On the other hand, since any brain region is connected with other regions and brain-wide networks, learning is characterized by modulations in connectivity between networks with different functions. Therefore, we hypothesized that learning performance is determined by functional connections among intrinsic networks that include both task-activated and less-activated networks. Subjects underwent resting-state functional MRI and a short period of training (80–90 min) in a working memory task on separate days. We calculated functional connectivity patterns of whole-brain intrinsic networks and examined whether a sparse linear regression model predicts a performance plateau from the individual patterns. The model resulted in highly accurate predictions (R2 = 0.73, p = 0.003). Positive connections within task-activated networks, including the left fronto-parietal network, accounted for nearly half (48%) of the contribution ratio to the prediction. Moreover, consistent with our hypothesis, connections of the task-activated networks with less-activated networks showed a comparable contribution (44%). Our findings suggest that learning performance is potentially constrained by system-level interactions within task-activated networks as well as those between task-activated and less-activated networks.