Research Abstract
短期時系列データを用いて非線形力学の因果関係を検出
Detecting Causality from Nonlinear Dynamics with Short-term Time Series
2014年12月12日 Scientific Reports 4 : 7464 doi: 10.1038/srep07464
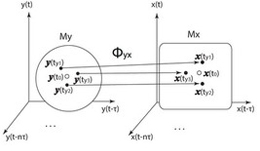
観測された時系列データから変数間の因果関係を定量化することは、さまざまな分野できわめて重要だが、特に観測されたデータ長が短い場合は困難な作業でもある。我々は、従来の方法と異なり、非線形力学系のアトラクターの埋め込み定理に基づき、きわめて短い時系列データだけからでも因果関係を検出できることを見いだした。すなわち、まずはじめに、2つの観測された変数間の交差写像の滑らかさの計量が因果関係の検出に使えることを示す。次に、この「交差写像の滑らかさ」(CMS: Cross Map Smoothness)を計算によって評価し、因果関係を推測するためのきわめて効率的なアルゴリズムを与える。このアルゴリズムは、非常に短い時系列データからでも高い精度を達成できる。最後に、さまざまなベンチマークからの数学的モデルと、生物系からの実際のデータの両方を使った分析で、我々の方法が有効であることを実証する。
Huanfei Ma, Kazuyuki Aihara & Luonan Chen
Corresponding Author(s)
Quantifying causality between variables from observed time series data is of great importance in various disciplines but also a challenging task, especially when the observed data are short. Unlike the conventional methods, we find it possible to detect causality only with very short time series data, based on embedding theory of an attractor for nonlinear dynamics. Specifically, we first show that measuring the smoothness of a cross map between two observed variables can be used to detect a causal relation. Then, we provide a very effective algorithm to computationally evaluate the smoothness of the cross map, or “Cross Map Smoothness” (CMS), and thus to infer the causality, which can achieve high accuracy even with very short time series data. Analysis of both mathematical models from various benchmarks and real data from biological systems validates our method.