計算機科学:人工知能がコンピューターチップの設計をスピードアップ
Nature
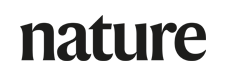
機械学習ツールを使用すると、コンピューターチップの設計が大幅に加速される可能性があることを報告する論文が、今週、Nature に掲載される。今回の研究では、この方法によって、人間のエンジニアによるチップ設計と同等以上の性能を発揮する実行可能なチップ設計が生成され、設計過程の所要時間はこれまでの数か月から数時間まで短縮されることが実証された。この方法は、グーグル社の人工知能コンピューターシステムの次世代モデルの設計にすでに用いられている。
コンピューターチップ上に種々のコンポーネントを配置する作業(フロアプランニング)は、チップ全体の性能を決定するカギである。コンピューターチップの物理的レイアウトの設計は、複雑で時間のかかる作業で、自動化が難しく、熟練した設計エンジニアの多大な努力を必要としていた。今回、Azalia Mirhoseini、Anna Goldieたちの研究チームは、機械学習ツールを用いて、フロアプランニングに要する時間を一気に短縮できることを実証した。
今回、Mirhoseiniたちは、チップのフロアプランニングを強化学習問題として提示し、実行可能なチップ設計を生み出すニューラルネットワークを開発した。具体的には、Mirhoseiniたちは、強化学習エージェントを訓練して、フロアプランニングをゲームとして扱うようにした。このゲームでは、コンピューターチップのコンポーネントが「ピース」、コンポーネントが配置されるキャンバスが「ボード」であり、チップ上のコンポーネント配置1万例が登録された参照データセットに基づく一連の評価指標によって定められる最適な性能を実現できれば「勝利者」になる。Mirhoseiniたちは、この方法により、専門家が設計したものと同等以上の実行可能なチップのフロアプランを6時間未満で生成でき、それぞれの新しい世代のコンピューターチップの生成に要する人間の労力を数千時間節約できる可能性があると指摘している。
同時掲載のNews & Viewsでは、Andrew Kahngが「現行の方法よりも優れていて、所要時間が短縮され、コストの削減された自動化チップ設計法が開発されたことで、チップ技術における『ムーアの法則』(1枚のチップ当たりのコンポーネントの数が2年でほぼ倍増するという法則)を示す曲線の軌道が維持される方向に働くだろう」と指摘している。また、Kahngは、Mirhoseiniたちのフロアプランのソリューションがグーグル社の次世代AIプロセッサーの設計にすでに用いられているという意外な新事実は、このチップ設計が大量生産に十分対応できるほど優れていることを実証している、と付言している。
Computer chip design can be greatly accelerated by using machine learning tools, according to a paper in Nature this week. The approach is shown to generate viable chip designs that perform at least as well as those produced by human engineers, but with the design process taking hours rather than months. This method is already being used in the design of the next generation of Google’s artificial intelligence computer systems.
The placement of different components on a computer chip is key to determining overall chip performance. Designing the physical layout of a computer chip is a complex and time-consuming task that has been difficult to automate, requiring intense efforts by expert human design engineers. Machine learning tools can be used to accelerate this so-called floorplanning process, Azalia Mirhoseini, Anna Goldie and colleagues demonstrate.
The authors pose chip floorplanning as a reinforcement learning problem and develop a neural network that can generate viable chip designs. They train a reinforcement learning agent to treat floorplanning as a game, in which the components are ‘pieces’, the canvas on which components sit is the ‘board’ and the ‘winning result’ is optimal performance according to a range of evaluation metrics (based on a reference dataset of 10,000 chip placements). The method can generate viable chip floorplans that are comparable or superior to those designed by human experts in under six hours, potentially saving thousands of hours of human effort for each new generation of computer chips, the authors note.
“The development of methods for automated chip design that are better, faster and cheaper than current approaches will help to keep alive the ‘Moore’s law’ trajectory of chip technology,” (in which the number of components per chip has roughly doubled every two years) writes Andrew Kahng in an accompanying News & Views article. He adds that the revelation that the authors’ floorplan solutions are being used in the design of Google’s next-generation AI processors demonstrates that the designs are good enough to be manufactured on a large scale.
doi: 10.1038/s41586-021-03544-w
「Nature 関連誌注目のハイライト」は、ネイチャー広報部門が報道関係者向けに作成したリリースを翻訳したものです。より正確かつ詳細な情報が必要な場合には、必ず原著論文をご覧ください。