計算機科学:過去の状態に立ち返る手法で複雑な課題を解決するAI
Nature
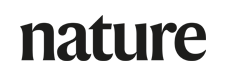
アタリ社の「モンテスマの復讐(Montezuma’s Revenge)」や「ピットフォール(Pitfall)」などの古典的なビデオゲームで、人間のプレイヤーや最先端の人工知能システムよりも高いスコアを達成する強化学習アルゴリズムのファミリー「Go-Explore」について報告する論文が、今週、Nature に掲載される。このアルゴリズムは、複雑な環境をよりよく探索するための方法になっており、真にインテリジェントな学習エージェントの作成に向けた重要な一歩となる可能性がある。
強化学習は、人工知能システムが、複雑な環境を探索し理解した上で決定を下し、最適な報酬の獲得法を学習できるようにするためのトレーニングに使うことができる。この報酬には、ビデオゲームでロボットが特定の場所に到達することや1つのレベルを完了することなどがある。しかし、既存の強化学習アルゴリズムは、フィードバックがほとんど得られない複雑な環境では悪戦苦闘すると考えられている。
今回、Adrien Ecoffet、Joost Huizingaたちの研究チームは、効果的な探索を実現する上での主な2つの課題を突き止め、これらの課題に対処するアルゴリズムのファミリーであるGo-Exploreを発表した。Go-Exploreは、環境を徹底的に探索し、環境内の位置関係を記憶するために役立つアーカイブを構築して、有望な中間段階や良好な結果(報酬)に至る経路を忘れないようにする。今回の研究では、これまで突破できなかったAtari 2600のゲームを全て攻略するためにGo-Exploreを使って、その可能性を実証した。Go-Exploreは、Montezuma’s Revengeでの過去最高スコアの4倍をマークし、Pitfallでは人間のプレイヤーの平均パフォーマンスを上回った(ちなみに、従来のアルゴリズムはPitfallで1点も得点できなかった)。さらに、Go-Exploreは、ロボットアームで物体を拾い上げ、4つの棚のいずれかに置かなければならず、そのうちの2つの棚が掛け金の掛かったドアの奥に設置されているというロボットタスクのシミュレーションも突破した。
Ecoffetたちは、有望な探索領域を記憶しておいて、そこに立ち戻るという単純な原理は、強力で一般的な探索手法であることを指摘した上で、今回の論文で発表したアルゴリズムをロボット工学、言語理解、薬剤設計に応用できるという考えを示している。
A family of reinforcement learning algorithms that score higher than human players and state-of-the-art artificial intelligence systems at classic Atari video games, such as Montezuma’s Revenge and Pitfall, is reported in this week’s Nature. Collectively known as Go-Explore, the algorithms offer a way to improve the exploration of complex environments, which may be an important step towards creating truly intelligent learning agents.
Reinforcement learning can be used to train artificial intelligence systems to make decisions by exploring and understanding complicated environments, and to learn how to optimally acquire rewards. Rewards may include a robot reaching a specific location or completing a level in a video game. However, existing reinforcement learning algorithms seem to struggle when complex environments offer little feedback.
Adrien Ecoffet, Joost Huizinga and colleagues identify the main impediments to effective exploration and present a family of algorithms that addresses these two challenges. Go-Explore can thoroughly explore environments and it builds up an archive to help it to remember where it has been, ensuring that it does not forget the route to a promising intermediate stage or successful outcome (the reward). The authors demonstrate the potential of the family of algorithms by using them to solve all previously unsolved Atari 2600 games. Go-Explore quadruples previous scores on Montezuma’s Revenge and surpasses average human performance on Pitfall (where previous algorithms were unable to score any points). Go-Explore can also solve a simulated robotic task where a robot arm must pick up an object and put it on one of four shelves, two of which are behind latched doors.
The simple principles of remembering and returning to promising areas for exploration are a powerful and general approach to exploration, the authors note. They suggest that the algorithms presented here could have applications in robotics, language understanding and drug design.
After the embargo ends, the full paper will be available at: https://www.nature.com/articles/s41586-020-03157-9
doi: 10.1038/s41586-020-03157-9
「Nature 関連誌注目のハイライト」は、ネイチャー広報部門が報道関係者向けに作成したリリースを翻訳したものです。より正確かつ詳細な情報が必要な場合には、必ず原著論文をご覧ください。