【地球科学】余震のパターンを予測する
Nature
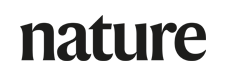
機械学習を用いて、大地震後の余震の発生位置を予測するための、応力ベースの判定基準を同定する研究について報告する論文が、今週掲載される。
余震は、大地震がもたらした地震応力の変化に対する応答であり、その規模と発生頻度を説明する経験則が存在している。しかし、余震の発生位置を説明し予測するのはより難しい。これまでの研究では、クーロン破壊応力の変化(地震発生時の応力の周辺物質への伝達に基づく)という因子を使って余震の発生位置を説明していたが、その適用可能性については論争がある。
今回、Phoebe DeVriesたちの研究グループは、13万1000組以上の地震と余震の組み合わせのデータを使ってニューラルネットワークを訓練した。このニューラルネットワークを使って、別の独立した3万組以上の地震と余震の組み合わせのデータについて調べたところ、余震の発生位置のパターンをクーロン破壊応力の変化よりも正確に同定し説明できることが示された。DeVriesたちは、今回の知見から、深層学習によって余震の予測がいかに改善され、地震誘発機序の解明への手掛かりがもたらされ得るかが明確になったと主張している。
A machine learning approach has been used to identify a stress-based law that can forecast the pattern of aftershock locations following large earthquakes. The findings are reported in this week’s Nature.
Aftershocks are a response to seismic stress changes generated by large earthquakes, and empirical laws exist that describe their size and frequency. However, explaining and forecasting the location of aftershocks has proved more difficult. Previously, a factor called Coulomb failure stress change (based on the transfer of stress during an earthquake to surrounding material) has been used to explain aftershock locations, but its applicability has been disputed.
Phoebe DeVries and colleagues trained a neural network using data from more than 131,000 pairs of earthquakes and aftershocks. They found that their network was able to identify and explain the pattern of aftershock locations in an independent dataset of more than 30,000 earthquake-aftershock pairs more accurately than can Coulomb failure stress change. The authors argue that the findings highlight how deep learning approaches can lead to improved aftershock forecasts and provide insights into the mechanisms of earthquake triggering.
doi: 10.1038/s41586-018-0438-y
「Nature 関連誌注目のハイライト」は、ネイチャー広報部門が報道関係者向けに作成したリリースを翻訳したものです。より正確かつ詳細な情報が必要な場合には、必ず原著論文をご覧ください。